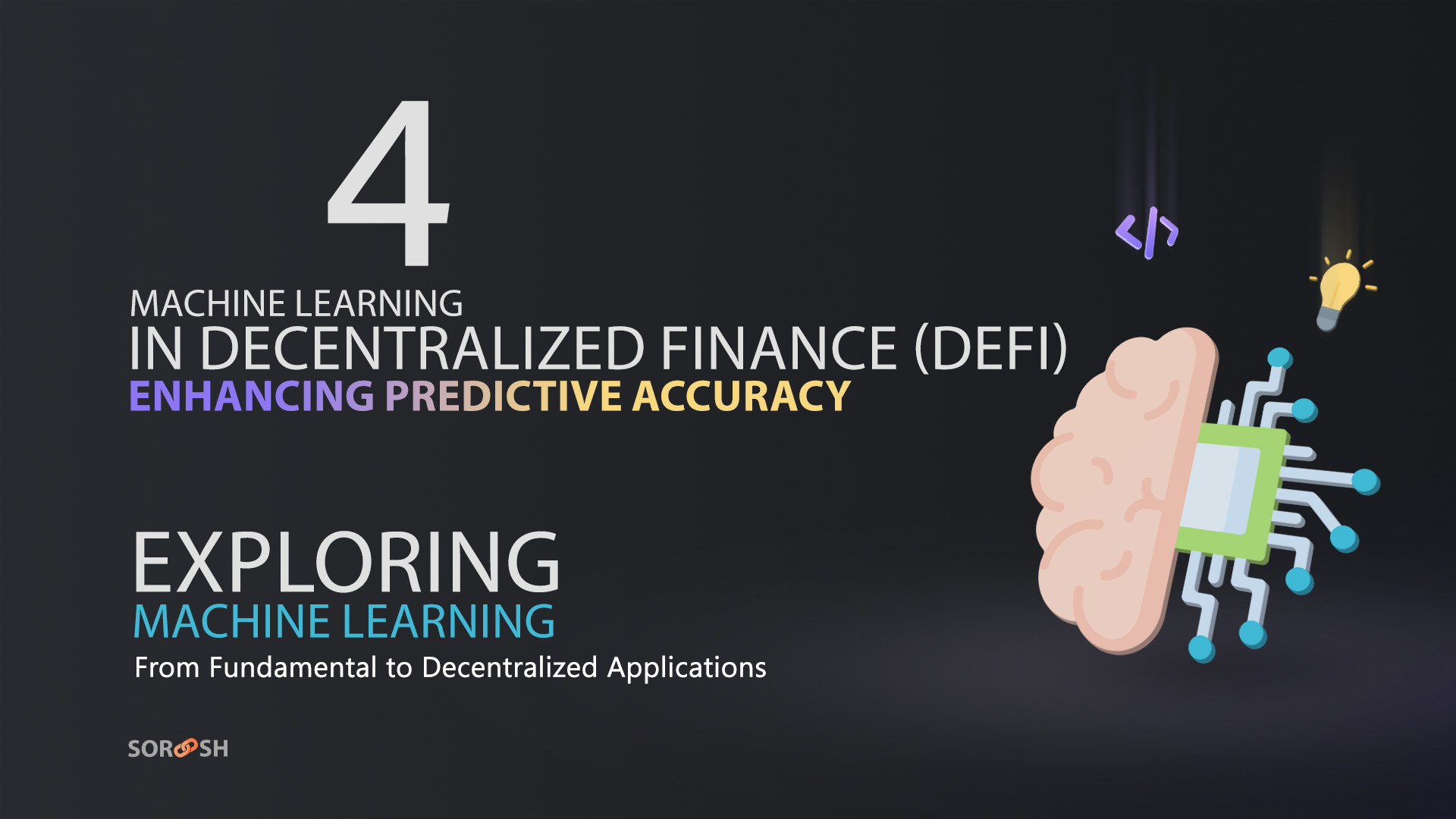
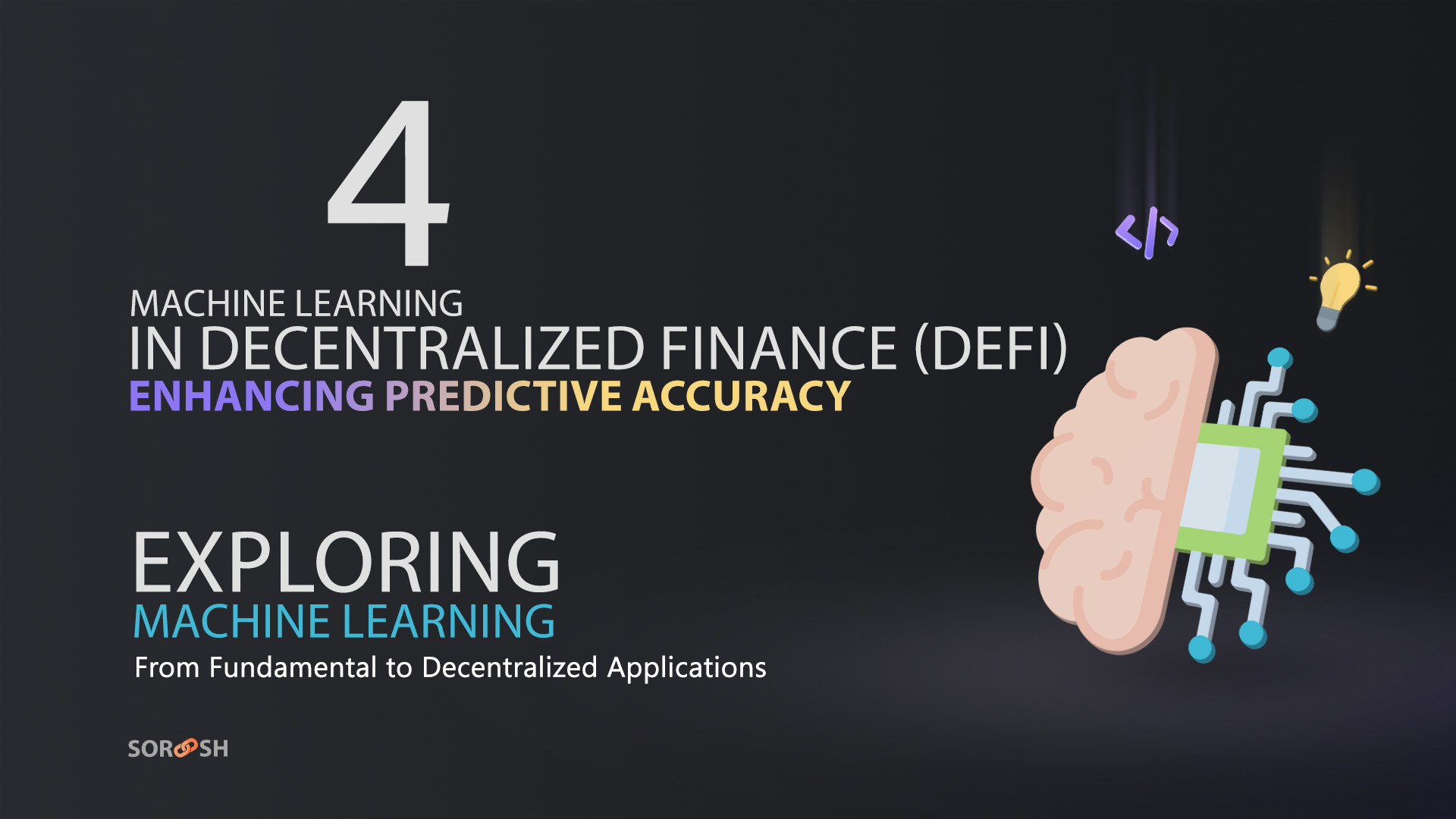
4.Machine Learning in Decentralized Finance (DeFi)
The fusion of machine learning (ML) with decentralized finance (DeFi) represents a thrilling advancement in fintech. This combination promises to revolutionize how we understand and interact with financial services, especially predictive modeling. In this blog post, we explore the role of machine learning in DeFi, the benefits it offers, the challenges it faces, and how it enhances predictive accuracy in financial operations.
What is Decentralized Finance (DeFi)?
Decentralized Finance, or DeFi, refers to a blockchain-based form of finance that does not rely on central financial intermediaries such as brokerages, exchanges, or banks to offer traditional financial instruments. Instead, it utilizes smart contracts on blockchains, most commonly Ethereum. DeFi platforms allow people to lend or borrow funds from others, speculate on price movements on a range of assets using derivatives, trade cryptocurrencies, insure against risks, and earn interest in savings-like accounts.
The Role of Machine Learning in DeFi
Machine learning in DeFi can be seen as a powerful tool to analyze, predict, and automate financial decisions. Here are some ways in which ML is currently being integrated into the DeFi space:
- Predictive Analytics: Machine learning models predict market trends, cryptocurrency price movements, and user behavior. This predictive capability can help risk assess, trade, and manage financial portfolios more effectively.
- Fraud Detection and Security: ML algorithms can analyze transaction patterns to detect unusual behavior that may indicate fraudulent activity. Such robust security measures are crucial in DeFi, where transactions are irreversible and anonymous.
- Personalized Financial Services: ML can help customize financial advice and product offerings to individual needs by analyzing user data and enhancing user engagement and satisfaction.
- Automated Trading and Investment: Algorithms can automate trading and investment decisions based on data-driven insights, reducing the need for manual intervention and potentially increasing transaction efficiency and profitability.
Enhancing Predictive Accuracy in DeFi
Predictive accuracy is critical in financial applications, directly impacting decision-making and profitability. In DeFi, machine learning enhances predictive accuracy through several mechanisms:
Data Handling Capabilities: ML algorithms can process and analyze vast amounts of unstructured data (from market data to social media sentiment) much faster and more accurately than human beings.
Real-Time Processing: Machine learning models can update predictions in real-time as new data arrives, which is essential in the fast-paced environment of DeFi markets.
Advanced Pattern Recognition: ML models excel in identifying complex patterns in data that would be unnoticeable to humans. This capability allows for more accurate forecasting of financial market trends and asset price movements.
Continual Learning: Machine learning models can improve with more data and feedback, increasing their accuracy and adaptability to new market conditions or assets.
Challenges Facing ML in DeFi
Despite the potential benefits, integrating machine learning into DeFi is not without challenges. These include:
Data Privacy and Security: Ensuring the privacy and security of sensitive financial data while utilizing ML models is a significant challenge, especially given DeFi's decentralized nature.
Model Transparency and Explainability: More transparent and understandable ML models are needed, as financial stakeholders require clear explanations of how models make their predictions.
Regulatory Compliance: Another hurdle that must be addressed is navigating DeFi's complex and evolving regulatory landscape while implementing ML solutions.
Conclusion
Machine learning has the potential to significantly enhance the efficiency, security, and profitability of decentralized finance. By improving predictive accuracy, ML allows DeFi platforms to offer more reliable and effective financial services. However, for this potential to be fully realized, the challenges of data privacy, model transparency, and regulatory compliance must be carefully managed. As we continue to witness the growth of DeFi, the integration of advanced machine-learning technologies will undoubtedly play a pivotal role in shaping its future.
Our next blog will delve into the fascinating intersection of blockchain technology and machine learning with "Training Machine Learning Models on the Blockchain: Potential and Pitfalls." We'll explore how blockchain, a technology that underpins cryptocurrencies like Bitcoin and Ethereum, can be leveraged to enhance the training of machine learning models. This integration promises increased transparency, security, and decentralization, but it's not without its challenges. From data privacy concerns to computational limitations, we will dissect both the groundbreaking potential and the significant obstacles that come with training ML models directly on the blockchain. Join us as we uncover the complexities and consider the future of machine learning in an increasingly decentralized digital landscape.
Brought to you by: Soroosh R&D team
Follow us on Social Media to get updates and announcements: